HGX Platform by NVIDIA is a groundbreaking advancement in AI and HPC. It has been designed to cater to the growing power demands of today’s data-intensive environments and merged with cutting-edge GPU technology; it delivers outstanding processing efficiency as well as flexibility. This introduction aims to give readers a preliminary insight into the incredible capabilities and implications of the NVIDIA HGX platform toward technological strides that set new performance and scalability benchmarks in AI and HPC projects.
What Makes NVIDIA HGX Essential for AI and HPC?
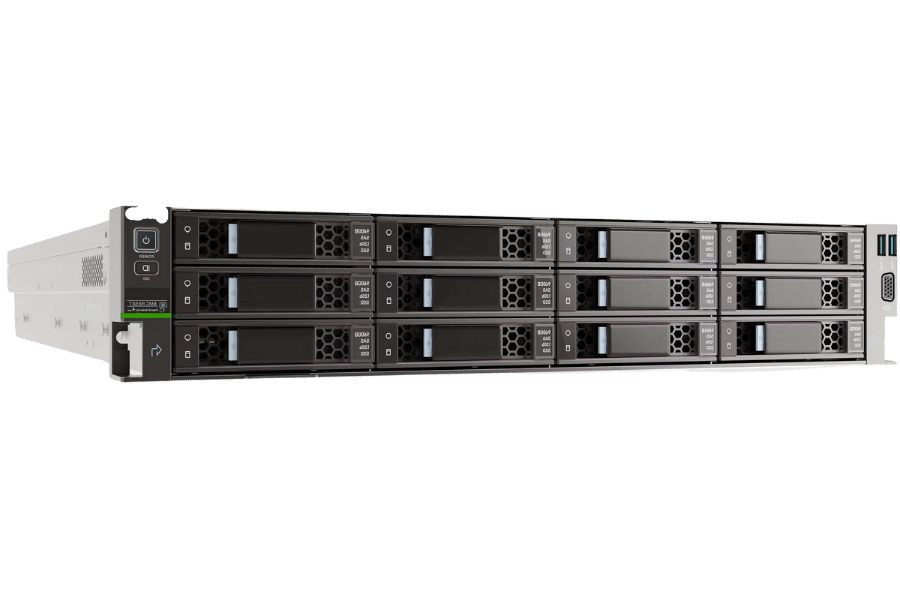
The Role of NVIDIA HGX in Advancing AI Research and Applications
The HGX model of NVIDIA is a stepping stone to advance AI research and many of its applications, providing breakthroughs that seemed impossible in the past. The platform possesses computational capability greater than any other, and developers and researchers dealing with AI can now process volumes of data more quickly than ever before. It is an important feature for training artificial intelligence systems on difficult cases like natural language processing, image recognition, and self-driving cars. An improved GPU technology enables more frequent testing, hence faster development of AI functionalities that can be applied in real-world situations via the HGX platform.
How NVIDIA HGX H100 and A100 GPUs Revolutionize HPC Workloads
NVIDIA HGX platforms, which feature the H100 and A100 GPUs, change HPC workloads by providing incomparably high performance and improved efficiency. The A100 GPU uses it’s Tensor Core technology to speed up various computing tasks like AI, data analytics, and scientific computation. Some of its outstanding features include Multi-Instance GPU (MIG) that enhances utilization as well as ensures workload isolation.
On the other hand, the HGX H100 GPU introduces a subsequent epoch in the history of GPU architecture specifically designed for exascale-based AI and HPC workloads. This builds upon what is presented in A100 to deliver superior performance through technologies such as Transformer Engine for AI operations and FP8 precision for faster calculation. Moreover, H100 brings in a new paradigm of processing big data patterns: The Hopper architecture making it among the most suitable solutions for pioneering AI investigations or demanding supercomputing applications.
Comparing NVIDIA HGX Platforms: The HGX A100 vs. HGX H100 Architecture
Contrasting the NVIDIA HGX platforms, HGX A100 and HGX H100’s unique architectural improvements unveil their respective roles in pushing the limits of AI and HPC capabilities. Here are some key differences.
- Computational Units: It is equipped with Ampere architecture Tensor Cores and CUDA cores that are optimized for AI and HPC workloads. On the other hand, Hopper architecture takes a step forward by having more Tensor Cores plus CUDA cores for much more computational throughput.
- Memory and Bandwidth: A100 has significant memory capacity and bandwidth improvements over previous generations, while the H100 further enhances this feature with higher capacity HBM3 memory and even greater bandwidth, enabling faster data processing and larger models
- AI Specific Features: While MIG, third-generation tensor cores were pioneered by A100, this progress is built upon by other features such as Transformer Engine that is custom-made for next-gen AI workloads like complicated language models introduced in the case of H100.
- Energy Efficiency: Energy efficiency is given equal priority on both platforms; however, the H100 incorporates new technologies intended to deliver unrivaled performance per watt, which is crucial when scaling up sustainable HPC and AI infrastructures.
To recapitulate, NVIDIA HGX H100 represents a substantial advancement in technology that builds on what A100 can do to address future demands of artificial intelligence (AI) as well as high-performance computing (HPC). Both have pivotal positions within their niches since they are dependent on user needs as determined by specific performance, scalability, and efficiency requirements, among others.
Exploring the Compute Power of NVIDIA HGX: A100 and H100 GPU Deep Dive
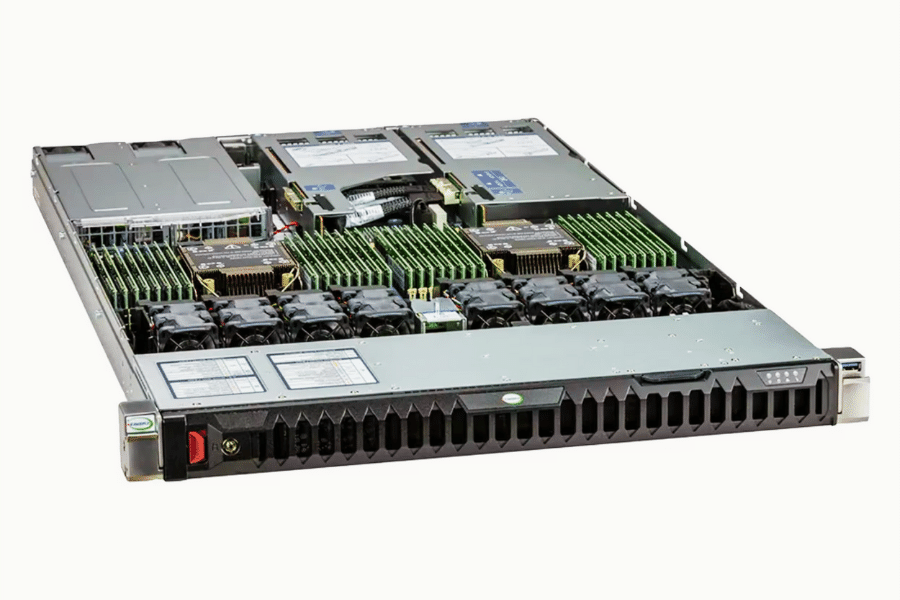
Understanding the Compute Capabilities of NVIDIA HGX H100 GPUs
The NVIDIA HGX H100 GPU is a breakthrough in GPU technology, especially for AI and HPC applications. Its design is based on the innovation of a Hopper architecture, which makes it possible to solve complex computational tasks with unmatched efficiency. Most notably, its more improved computing capacities are seen in the following:
- AI and Machine Learning: The H100 is really good at accelerating AI and machine learning loads because of its better Tensor Cores and CUDA cores count than other products available in the market. These cores are optimized for deep learning computations, hence making the H100 perfect for training complicated neural networks and processing huge datasets.
- High-Performance Computing (HPC): For this purpose, researchers can solve complex scientific or engineering problems faster due to the advanced architecture of the H100. It stems from the increased computational throughput of this GPU as well as the ability to manipulate large amounts of data all at once.
NVIDIA A100 in the HGX Platform: Accelerating a Broad Range of AI and HPC Applications
NVIDIA’s A100 was a predecessor to the H100. It set an extremely high standard in computing, making it a versatile accelerator for both AI and HPC workloads. Some of its major contributions include:
- Versatility: Built for tasks ranging from AI inference to high-fidelity simulations on the A100 architecture which is very flexible in nature. This implies that there can be superior performance among various tasks without any specialized hardware.
- Scalability: By supporting NVLink and NVIDIA’s SXM form factor, the A100 permits creation of large, powerful GPU clusters. While addressing the most computationally demanding AI and HPC projects this feature of scalability becomes vital.
Key Features and Benefits of NVIDIA HGX’s SXM5 and NVLink Technology
NVIDIA’s Scalable Link Interface (SXM5) and NVLink technology are vital in supporting the extraordinary performance and efficiency of the HGX platform. These advantages include:
- Faster Data Transfer Speeds: The NVLink technology acts as a high-speed data transfer path between GPUs, thus significantly reducing communication overheads. This is most critical in applications that need to exchange data across GPUs, often like distributed deep learning.
- Increased Power Efficiency: The SXM5 form factor has been specifically designed to optimize power use, hence having the H100 and A100 GPUs working at peak performance but not consuming too much electricity. Thus, this design consideration is very important for constructing sustainable, massive computational infrastructures.
- More Bandwidth: When combined, NVLink and SXM5 offer a higher bandwidth connection than traditional PCIe interfaces. This extra bandwidth is vital in order to feed the GPU with more data at faster rates enabling them to perform very fast throughput computing operations.
To sum up, the NVIDIA HGX H100’s compute capabilities together with what A100 does for this platform highlight NVIDIA’s devotion towards progressing GPU technologies. Through SXM5 and NVLink force, these GPUS provide unmatched productivity, scalability, resourcefulness hence they play an active role in setting new benchmarks for AI as well as HPC deployments.
Accelerating Server Platforms for AI: Why NVIDIA HGX Stands Out
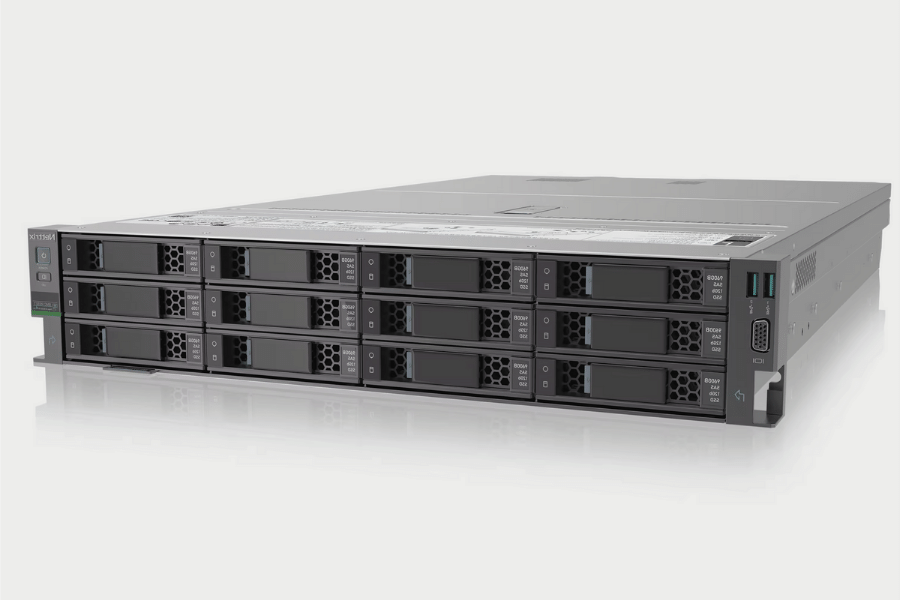
The adoption of NVIDIA HGX for server platforms targeting artificial intelligence (AI) offers a number of key benefits that directly affect the performance and efficiency of AI applications. Various case studies demonstrate the application of NVIDIA HGX in actual AI data centers and its use in generating scalable, high-performing AI infrastructures, demonstrating the huge contributions it has made to the industry.
- Scalability: NVIDIA HGX architecture is highly scalable, making data centers capable of effectively handling increasing demands for AI workloads. Such scale makes it possible to seamlessly meet computational needs in current and future AI projects without completely revamping the infrastructure.
- Performance: The specially designed GPUs with NVLink and SXM5 improve their performance significantly. They deliver faster processing and transfer of data required for training complex models of AI as well as running time-sensitive algorithms with less latency.
- Energy Efficiency: The power-efficient design of this module reduces overhead costs associated with high-performance computing tasks. This efficacy is important for sustainable growth in AI research and deployment making NVIDIA HGX an eco-friendly option for any data center.
- Case Studies – Real-world Impact: Different industries have benefited from the implementation of NVIDIA HGX platforms. For instance, in healthcare, accelerated medical image analysis by HGX-based systems improved diagnostic speed and accuracy. In autonomous vehicle development, processing massive amounts of data needed for training and testing self-driving algorithms has been made easier, thus leading to new technologies being taken to market more quickly through the use of HGXs.
- Role in AI Infrastructures: With respect to building efficient AI infrastructures supporting high-performance workloads, it acts as a pillar upon which everything else rests. While this architecture is created around individual project requirements, NVidia designed it as a tool for fostering innovation within Ai by providing a platform capable of adjusting itself according to changes taking place across Ai technologies
To summarize, incorporating NVIDIA HGX into server platforms results in breakthroughs in AI research and applications at AI-focused data centers. In fact, its unrivaled scalability, performance, and energy efficiency make it a must-have for future AI technology development.
Deploying NVIDIA HGX in Data Analytics, Simulation, and Deep Learning
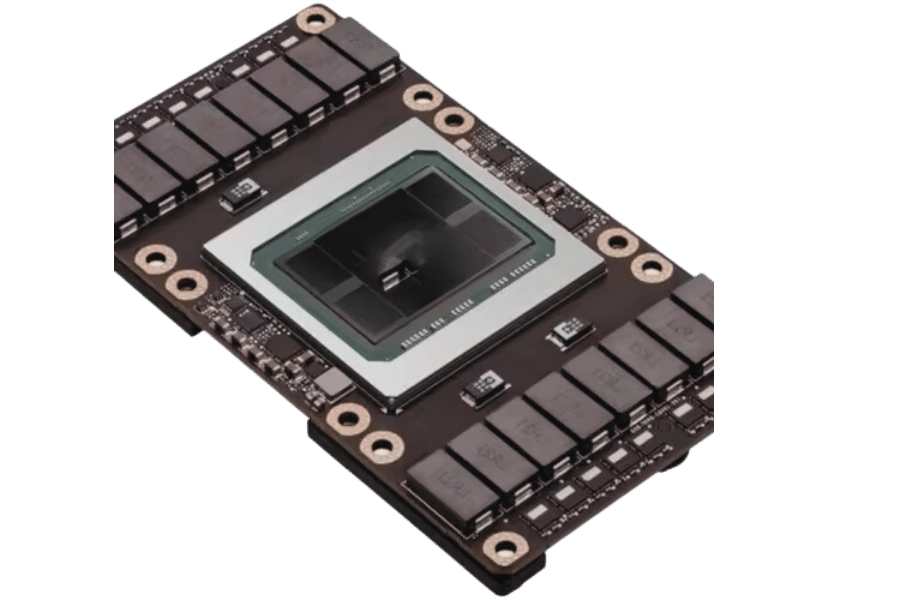
Accelerating Data Analytics with NVIDIA HGX
NVIDIA HGX platforms are very good at speeding up data analytics, enabling businesses and researchers to make quick out of huge datasets. By utilizing GPU technology, which is parallel processing in nature, HGX can significantly reduce the time it takes to process data. This acceleration is usually important for making decisions on time and also getting ahead in various industries.
- Parallel Computing Power: Compared to traditional CPU-based analysis, GPUs perform multiple calculations at the same time, resulting in a manifold increase in data analytic workflows.
- Optimized Software Ecosystem: NVIDIA offers a wide range of optimized software tools and libraries, such as RAPIDS, designed specifically for accelerated data processing on GPUs. This ecosystem can easily integrate into popular data analytics frames, thereby increasing their performance without extensive code modifications.
- Scalability: The modular design of the HGX platforms enables scaling based on data size and complexity, hence giving flexible and cost-effective ways of handling any scale of analytics workloads.
The Role of NVIDIA HGX in Simulation and Modeling
Simulation and modeling are indispensable in the world of scientific advancement, while NVIDIA HGX’s computational capabilities play a central role in these activities.
- HPC Capabilities: HGX boasts enough computational power required for computations involving complex simulations like climate modelling, molecular dynamics and astrophysical simulations that need enormous computational resources.
- Accuracy and Swiftness: The precision provided by the Tensor Cores of NVIDIA along with the fast GPUs they have makes simulation and models that can be more effectively executed at higher fidelity over shorter durations. This improves the accuracy of scientific predictions as well as technology development.
- AI-Boosted Simulations: AI integration into traditional simulation workflows is also made possible by HGX, resulting in better predictions, optimizations, and findings in scientific research.
Enhancing Deep Learning with NVIDIA HGX Platforms
The deep learning applications benefit much from NVIDIA HGX platforms’ computational prowess as they handle large volumes of data and hard-to-manage neural networks.
- Faster Training: Deep Learning models, especially those that have huge neural networks, require a lot of computing power to train. In this way, HGX speeds up the process such that training time is reduced from weeks to days or even hours.
- Increased Complexities and Sizes of Models: The greatness of HGX is in its ability to create more elaborate models through which AI has advanced beyond some computer limitations.
- Energy Efficiency: For all their mightiness, the HGX platforms are designed with energy efficiency in mind; without it there can be no sustainable development for AI, especially when it entails training large models that can consume significant amounts of energy.
In conclusion, NVIDIA HGX acts as a key accelerator for data analytics processes, simulations, and scientific models while improving deep learning via exceptional computational powers. Its combination of scalability, efficiency, and design contributes greatly to innovation across different sectors.
The Future of AI and HPC with NVIDIA HGX: 8-GPU and 4-GPU Configurations
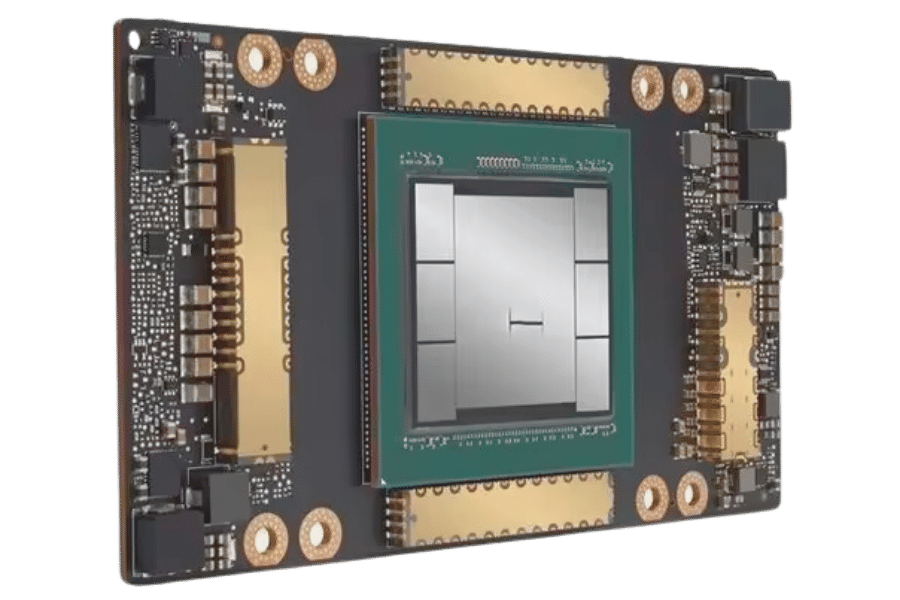
Exploring the Potential of 8-GPU and 4-GPU NVIDIA HGX Configurations for AI and HPC
NVIDIA HGX platform is available in two configurations, one with eight graphics processing units (GPUs) and the other with four GPUs. They capture the power of many optimized for parallel processing by multiple GPUs, which can be very helpful in processing large data sets and complex algorithms within a shorter period of time.
- Scalability as well as Performance: In terms of scalability and performance, this 8-GPU configuration is unrivaled because it can cater to the requirements of complex AI models and HPC simulations that are demanding to run. It enables maximum parallelization capacity, which is crucial for deep learning, scientific computing, and 3D rendering. On the other hand, a 4-GPU setup offers a cost-effective approach while still maintaining relatively high computational powers, especially useful in circumstances where mid-range applications are involved.
- Cost Effectiveness: These arrangements integrate more GPUs into one platform, thus reducing the need for multiple servers, hence saving on the space occupied as well as power consumption. For organizations intending to scale their business operations yet ensure manageable running costs this aspect is vital.
- Versatility plus Adaptability: The two configurations are suitable for diverse scientific investigations or research across various domains like molecular modeling, computational genomics, financial simulations, etc. Their flexibility allows HGX to provide continuing support without requiring major changes in hardware should new needs emerge.
The Future Role of NVIDIA HGX in AI and HPC
NVIDIA HGX, the spearhead of GPU server platforms’ evolution, has been setting new standards in AI and HPC. The scalability, efficiency, and performance of HGX platforms will become more important as computational demands increase exponentially. Their contribution is not only to this generation’s AI models and HPC applications but also to future innovations that will require even greater processing power.
How NVIDIA HGX is Shaping the Next Generation of AI Models and HPC Applications
NVIDIA HGX is a leader in empowering future AI models and HPC applications by:
- Advanced Architectural Features: The introduction of special AI hardware features like Tensor Cores, together with mixed-precision computing support, makes computations faster and more energy-efficient.
- Enhanced Data Processing Capabilities: Increased throughput and reduced latency enable the HGX platform to handle larger datasets, allowing for more detailed and accurate models and simulations.
- Cross-Industry Innovation: NVIDIA HGX’s flexibility and power have already been demonstrated in various sectors that have seen innovation in Autonomous vehicles, climate change models, drug discovery, etc.
In conclusion, the 4-GPU and 8-GPU configurations of the NVIDIA HGX platform are a major leap forward in computing technology, offering researchers, scientists and engineers the tools they need to address contemporary challenges while exploring future possibilities. Their impact can be felt at every level of scientific discovery up to technological innovation hence their role cannot be understated when it comes to shaping AI/ HPC future.
NVIDIA HGX’s Impact on AI Training and Inference: A New Era of Computing
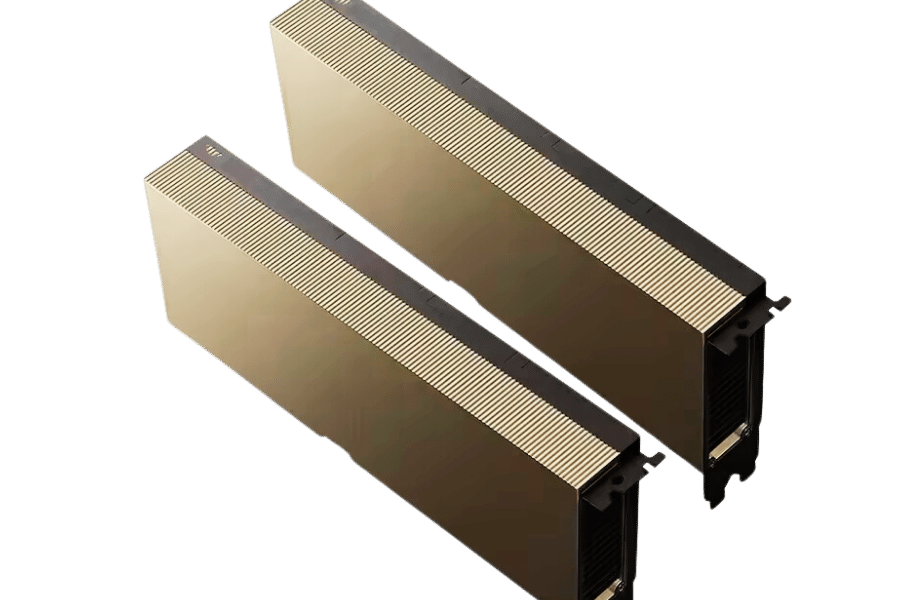
Advancements in AI Training with NVIDIA HGX’s Powerful Computing Capabilities
The rapid advancement of artificial intelligence (AI) training via NVIDIA HGX’s powerful computational infrastructure represents an important watershed in the development and improvement of models. This is particularly crucial for deep learning models that consume vast amounts of computation to analyze large datasets. Some of the notable improvements on NVIDIA HGX include:
- Multi-GPU Scalability: By using NVIDIA NVLink and NVSwitch technology, HGX platforms enable high-speed connections between GPUs and allow for scalable training workloads which were not possible before.
- Optimized Tensor Cores: Made explicitly for AI workloads, these cores speed up matrix operations, a basic element in deep learning algorithms hence drastically accelerating the training period.
- Mixed-Precision Computing: With FP32, FP16 and INT8 precision formats supported by HGX, models can be trained faster with no accuracy loss thus making it time-efficient as well as energy-efficient.
The Role of NVIDIA HGX in Accelerating Inference and Real-time AI Applications
The inference territory is notable for NVIDIA HGX because it enables AI applications, some aspects of which include natural language processing and image recognition, to make immediate decisions. Its architecture is designed to minimize latency and increase throughput for real-time applications. This involves the use of NVIDIA TensorRT and mixed-precision computing, which:
- Reduces computational overhead: It lowers the time taken to process new data, hence ensuring that AI apps can respond in real-time.
- Improves Throughput: It allows for a larger number of inferences processed at the same time, thus essential for live environments that need to handle large volumes of data.
Envisioning the Future: How NVIDIA HGX Continues to Redefine the Landscape of AI and HPC
NVIDIA HGX is going to carry on its journey of remolding the field of Artificial Intelligence (AI) and High-Performance Computing (HPC). It is constantly changing with time and moving towards a stage where it becomes impossible to have enough computational power for AI and HPC anymore. The progression forward may lead to:
- 1. More integration into new technologies: As quantum computing and edge AI technologies continue maturing, NVIDIA HGX will probably deepen its connection with these platforms, enabling much more powerful calculations.
- 2. Focus on sustainability: Future HGX versions will likely be designed with greater energy concerns in mind without compromising on performance.
- 3. Customization for specific AI applications: The optimization of NVIDIA HGX platform would enable it to handle industry-specific artificial intelligence tasks thus achieving best results under different workloads.
In short, the NVIDIA HGX is not simply a technology that contributes to development in AI and HPC but actually makes it happen from an impossibility perspective, transforming how technology will look in the days ahead.
Reference sources
- NVIDIA Official Website – NVIDIA HGX OverviewInformation regarding the NVIDIA HGX platform is available in full detail on NVIDIA’s official website. It has a lot of detailed specifications, architectural design principles, and descriptions of how it can be integrated within AI and high-performance computing environments. The foundational information about the NVIDIA HGX platform in this website is crucial for its understanding, including its role in enabling advanced scientific computing tasks and accelerating artificial intelligence workloads.
- IEEE Xplore Digital Library – Research on High-Performance Computing Enhancements via NVIDIA HGXIn one of the articles in the IEEE Xplore Digital Library, there is an empirical study that focuses on improved high-performance computing through the NVidia HGX platform. This peer-reviewed study deeply reflects upon the computational performance of this platform and how it influences delay in operating complex AI models and simulations. For readers to understand the NVIDIA HGX’s technical advancements in terms of practical applications, they have to read this article, which presents findings and discussions.
- TechCrunch – Analysis of NVIDIA HGX’s Role in the Future of AI and ComputingIn a critical analysis, TechCrunch explains the importance of the NVIDIA HGX platform within the larger context of AI evolution and high-performance computing in the next few decades. This article gives insights into how NVIDIA HGX is set to sustain escalating computational needs in artificial intelligence studies and build smart AI solutions with ever-increasing complexity. If you are looking to understand what market and technological trends have been influenced by NVIDIA HGX mean for AI futures or computing, this paper will be helpful for you.
Frequently Asked Questions (FAQs)
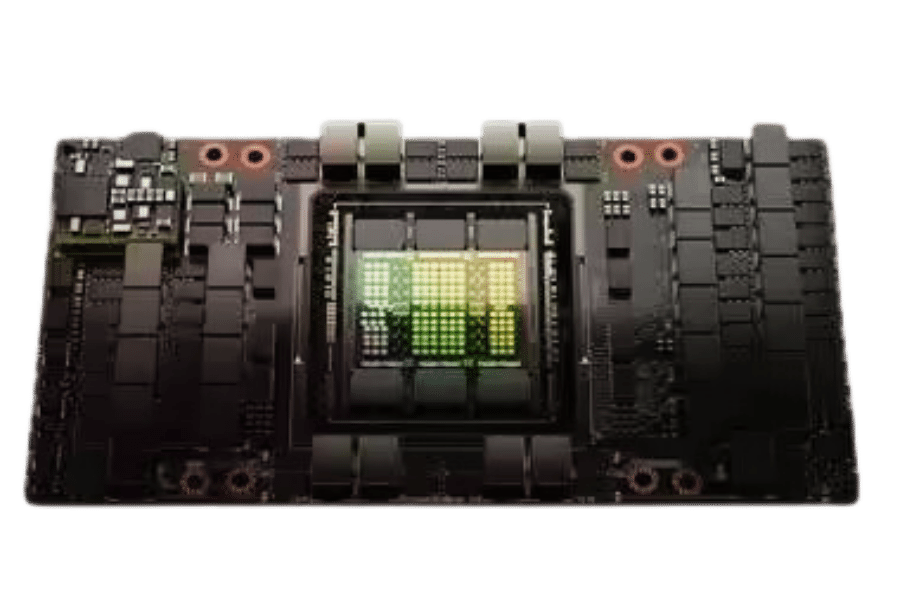
Q: What is the NVIDIA HGX Platform?
A: The NVIDIA HGX Platform is a computing platform made to accelerate AI (Artificial Intelligence) and HPC (High-Performance Computing) workloads. It has been designed to speed up today’s Da Vinci and Einsteins’ work via deep learning, scientific computing, and data analytics at unprecedented scales, leveraging powerful accelerated hardware such as eight H100 Tensor Core GPUs.
Q: How does the NVIDIA HGX Platform enhance computing performance?
A: Nvidia NVSwitch introduces high-speed interconnects between GPUs on a single node and across multiple nodes in the NVIDIA HGX Platform, thereby maximizing throughput while minimizing bottlenecks in data flow. This arrangement, coupled with NVIDIA InfiniBand and NVIDIA SHARP technology, improves computations within networks significantly, ensuring faster data transfer rates across nodes, which in turn boosts response time taken by AI and HPC queries.
Q: What components are included in the NVIDIA HGX platform?
A: These include new generation H100 SXM5 Tensor Core GPUs that provide a significant increase in terms of GPU memory and compute power for better performance. The NF5488A5 baseboard also provides advanced features like NVLink Switch that enables high-speed interconnects as well as InfiniBand for top-quality but low-latency networking, all of which ensure that the system can handle larger scale computational tasks more easily.
Q: Why is GPU memory important in the NVIDIA HGX Platform?
A: The importance of GPU memory cannot be underestimated since it facilitates storage as well as rapid computation of large datasets typically deployed in AI and deep learning models. For instance, having increased GPU memory on these H100 SXM5 GPUs allows dealing with much bigger models and datasets thus leading to reduced processing times as well as overall system efficiency when dealing with learning applications or those meant for AI.
Q: How does NVIDIA InfiniBand support the NVIDIA HGX Platform?
A: NVIDIA InfiniBand technology serves the NVIDIA HGX Platform by providing a high-performance networking option that allows for extremely low latency and high bandwidth communication between the system’s nodes. This is important for applications with rapid data exchange and synchronized operations executed across different nodes, thus accelerating complex, data-heavy AI research or HPC tasks.
Q: Can the NVIDIA HGX Platform be used for tasks beyond AI and HPC?
A: While primarily designed for accelerating artificial intelligence (AI) and high-performance computing (HPC) workloads, NVIDIA HGX Platform’s robust computing capabilities are positioned to address other applications that demand significant computational power, such as data analytics, scientific simulation, or deep learning model training involving natural language understanding, image recognition or next generation scientific research initiatives. This demonstrates its flexibility over traditional AI and HPC workloads.
Q: What makes the NVIDIA HGX Platform unique in handling exploding model sizes in AI?
A: By integrating the immense GPU memory and powerful compute resources of Nvidia’s H100 Tensor Core GPUs with the most advanced InfiniBand and NVLink networking technologies, this is how The NVIDIA HGX Platform deals with the issue of exploding model sizes in AI. Henceforth, it can process big data volumes within a short time interval in real-time, which also enables it to break barriers to artificial intelligence research using deep learning, which has always been seen as unattainable because of limitations on processing capacity and data transfer rates.
Q: How does the NVIDIA HGX Platform accelerate the work of Da Vinci and Einstein of our time?
A: In this regard, by providing an unbeatable platform leveraging state-of-the-art Graphics Processing Unit (GPU) technology, high-bandwidth connectivities, coupled with sizeable GPU memories, at once accelerating DGX station’s “parallel throughput” required for both Data preprocessing & Model training phases. Consequently, scientists, engineers, and researchers spend more time on creative problem-solving instead of routine manual tasks, enabling such DaVinci and Einstein characters to breed new paradigms in AI/Deep Learning / High-Performance Computing.
Q: What future advancements can we expect in NVIDIA HGX Platforms?
A: With the increasing demand for higher computation resources, categories of enhancements that could be anticipated from nVIDIA under their forthcoming HGX Platform iterations include greater computational speeds, improved data processing efficiency, and bigger GPU memory sizes. To meet these evolving needs, new GPU architectures will be introduced while network technologies such as the next generation of NVLink and InfiniBand will also be improved upon for better performance. Further, optimized software frameworks are expected to be developed in order to support this growing complexity inherent in HPC, Deep Learning, and AI applications.
Related Products:
-
NVIDIA MMA4Z00-NS400 Compatible 400G OSFP SR4 Flat Top PAM4 850nm 30m on OM3/50m on OM4 MTP/MPO-12 Multimode FEC Optical Transceiver Module $650.00
-
NVIDIA MMA4Z00-NS-FLT Compatible 800Gb/s Twin-port OSFP 2x400G SR8 PAM4 850nm 100m DOM Dual MPO-12 MMF Optical Transceiver Module $850.00
-
NVIDIA MMA4Z00-NS Compatible 800Gb/s Twin-port OSFP 2x400G SR8 PAM4 850nm 100m DOM Dual MPO-12 MMF Optical Transceiver Module $750.00
-
NVIDIA MMS4X00-NM Compatible 800Gb/s Twin-port OSFP 2x400G PAM4 1310nm 500m DOM Dual MTP/MPO-12 SMF Optical Transceiver Module $1100.00
-
NVIDIA MMS4X00-NM-FLT Compatible 800G Twin-port OSFP 2x400G Flat Top PAM4 1310nm 500m DOM Dual MTP/MPO-12 SMF Optical Transceiver Module $1200.00
-
NVIDIA MMS4X00-NS400 Compatible 400G OSFP DR4 Flat Top PAM4 1310nm MTP/MPO-12 500m SMF FEC Optical Transceiver Module $800.00
-
Mellanox MMA1T00-HS Compatible 200G Infiniband HDR QSFP56 SR4 850nm 100m MPO-12 APC OM3/OM4 FEC PAM4 Optical Transceiver Module $200.00
-
NVIDIA MFP7E10-N010 Compatible 10m (33ft) 12 Fibers Low Insertion Loss Female to Female MPO Trunk Cable Polarity B APC to APC LSZH Multimode OM3 50/125 $47.00
-
NVIDIA MCP7Y00-N003-FLT Compatible 3m (10ft) 800G Twin-port OSFP to 2x400G Flat Top OSFP InfiniBand NDR Breakout DAC $275.00
-
NVIDIA MCP7Y70-H002 Compatible 2m (7ft) 400G Twin-port 2x200G OSFP to 4x100G QSFP56 Passive Breakout Direct Attach Copper Cable $155.00
-
NVIDIA MCA4J80-N003-FTF Compatible 3m (10ft) 800G Twin-port 2x400G OSFP to 2x400G OSFP InfiniBand NDR Active Copper Cable, Flat top on one end and Finned top on other $600.00
-
NVIDIA MCP7Y10-N002 Compatible 2m (7ft) 800G InfiniBand NDR Twin-port OSFP to 2x400G QSFP112 Breakout DAC $200.00